Blog
April 29, 2025
The AI Maturity Model: Scaling AI from Pilot to Pioneering
AI,
Data Management,
Security & Compliance
Your organization may be one of the many that is rushing to implement AI. But do you know where you fall on the AI maturity model?
More than just a framework for understanding AI, the AI maturity model is a strategic guide that helps turn AI investments into tangible business results.
A 2024 IDC study commissioned by Microsoft titled “The Business Opportunity of AI” found that organizations gain a $3.7x return for every $1 spent on generative AI. But many organizations find it hard to move past pilot projects. While most early adopters thrive, they struggle to achieve real, scalable AI success.
Understanding where you fall on the scale can give your business an advantage over competitors. Let’s dive into what exactly the AI maturity model is, what defines the key levels, and some of the challenges of securely adopting AI.
First, What Is the AI Maturity Model?
The AI maturity model outlines progressive stages of AI adoption. This begins from initial experimentation to enterprise-wide integration. It helps businesses assess their capabilities, identify gaps, and scale AI in a way that maximizes impact.
Several businesses have differing views on what the AI maturity model (or “scale,” or “curve”) encompasses. Still, these maturity frameworks have similar goals. They aim to test AI capabilities, remove roadblocks, and plan a clear path for AI-driven growth.
Understanding where your organization stands with AI gives you useful standards to measure your progress and growth. This applies whether you're testing AI out with small projects or making it a central part of your business strategy.
Key Levels of the AI Maturity Model
As organizations incorporate AI into their daily work, they move through different growth stages. Not just in AI adoption, but in how they evolve their use of data. This journey mirrors a broader shift — from simply managing data, to extracting meaningful analytics, and ultimately enabling intelligence that drives innovation.
Again, experts don't all agree on one specific model for AI growth. But it helps to think about AI development as happening in three main levels: operational AI, strategic AI, and transformational AI. Each stage unlocks new opportunities and competitive advantages for organizations.
AI Maturity Level | Description | Key Focus Areas | Example Use Cases |
Level I Operational AI | AI automates repetitive tasks to boost efficiency. Most organizations remain at this stage and handle vast data without deep AI integration. | - Process automation (Robotic Process Automation, Natural Language Processing) - Data modernization - Cost reduction & efficiency | - Call center automation - AI-powered chatbots - Basic business process automation |
Level II Strategic AI | AI is embedded into business operations to drive revenue, improve decision-making, and enhance user experiences. Strong data governance is essential at this stage. | - Data security and compliance - Predictive analytics - AI-driven insights | - Financial forecasting - Customer personalization - Smart product enhancements |
Level III Transformational AI | AI is at the core of business strategy. It drives industry change through cognitive intelligence and deep learning. AI is also used to create new capabilities and reshape markets. | - Generative AI & deep learning - AI-powered products & services - AI ethics & responsible AI | - AI-curated content - AI-driven automation in business decision-making - Advanced AI-powered co-pilots and personalization |
As organizations advance through these three levels, they simultaneously advance through a data modernization journey.
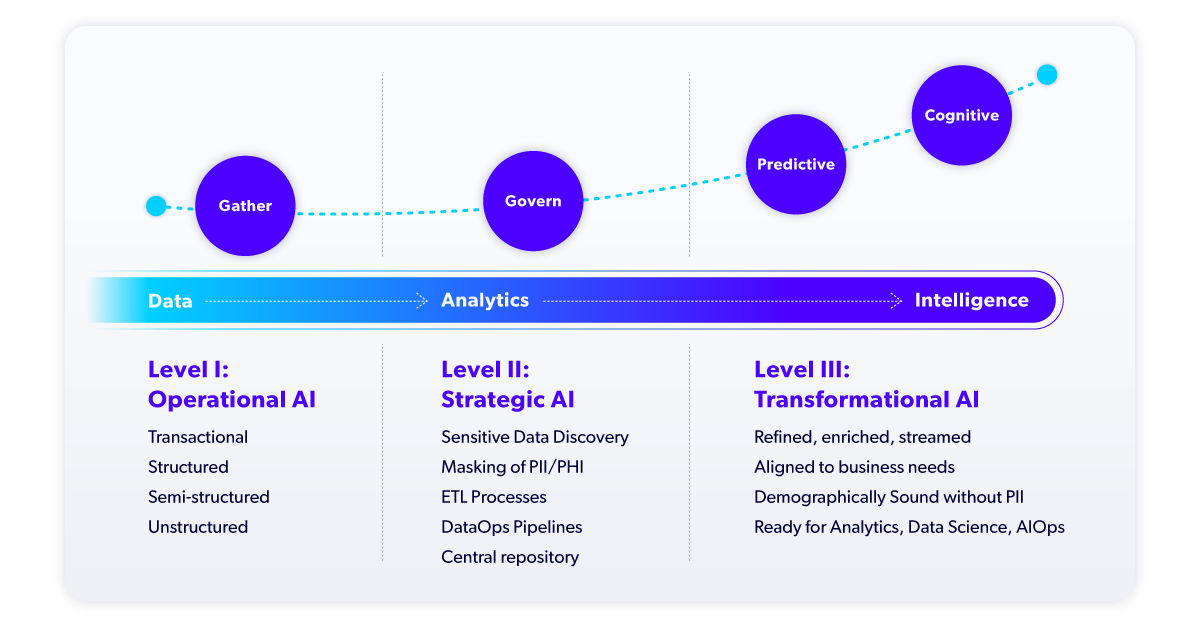
Level I: Operational AI
At this foundational level, organizations are doing business as usual (highly transactional and moderately analytical), with individuals or teams applying AI to drive operational efficiency. These "dabblers" use AI to automate repetitive tasks performed by human employees. Level I organizations should apply AI to internal opportunities with a clear cost-benefit analysis. Some examples include call center automation and AI services like agentic process automation to cut manual, repetitive work.
Most Level I organizations manage large amounts of structured and unstructured data. This includes customer records, transactional databases, and business operations. This is data with a ton of potential for AI, but only if used safely.
At this level, it's vital for organizations to build an AI governance model to enable innovation while ensuring customer privacy and cost controls. You don't want your employees "going rogue" with their own ChatGPT accounts, but you also want to harness the power of these platforms in a meaningful and safe way to move forward.
📘 Related reading: AI and Data Privacy: 3 Things You Need to Know
Level II: Strategic AI
With the right safeguards and practices, organizations in this level go beyond automation. They integrate AI across their business to drive significant revenue or earnings impact. AI enhances user experience and customer value. It also helps improve decision-making and creates smarter products and services.
A key component of this phase is data compliance, which ensures security and scalability. Key focus areas include:
Sensitive data discovery and data masking: Identifying and protecting personally identifiable information (PII) and protected health information (PHI).
DataOps pipelines: Managing data flow and integrity across enterprise systems.
AI-driven insights: Developing predictive models to forecast business outcomes.
A well-managed data system helps organizations use predictive analytics. This gives them deeper insights and prepares them for the next stage of AI growth.
The governance is in place, the stage is set. This is the "gateway" to enterprise intelligence. Let me make this clear: you CANNOT skip this step.
Good compliance practices will ensure you can train and improve AI projects with standard CI/CD principles including shift-left and continuous testing, while also protecting sensitive customer data.
Level III: Transformational AI
At the highest stage, organizations use AI to drive industry change and innovation. These visionary companies embed AI into their core products and services, driving innovation in the market.
The following are some real-life examples of industries using transformational AI:
Financial services create fully autonomous banking and hyper-personalized advisory services.
Healthcare shifts to predictive medicine and precision treatments.
Insurance develops dynamic risk models and instant claims processing.
Retail reimagines supply chains and blends physical-digital experiences.
At this stage, businesses are moving into an area just beyond traditional analytics. Forecasting, regression modeling, and prediction start coming into the picture here. This unlocks both business intelligence (internal to the organization) and personalization (which is highly desired among customers).
One key thing to remember is that there's a difference between traditional machine learning (algorithmic) models, and modern generative AI (algorithmic but with net new creation).
Due to the generative nature of LLMs, diffusion models, etc., there are new challenges to look out for:
Models learning real data they cannot forget
Models learning real data they may accidentally share with other users
Hallucinations of critical details that can impact your business (internal) or customers (external)
Worried about AI and Data Privacy? You’re Not Alone.
82% of respondents in the latest State of Data Compliance and Security Report expressed concerns about AI and data privacy.
Data security is critical no matter which stage you are in on the AI maturity scale. AI doesn’t forget the data it is trained on. If it ingests sensitive data, that data can reappear in unexpected and uncontrollable ways.
My eBook, “AI Without Compliance,” offers a primer on what leaders are up against as their teams adopt AI. It explores:
- What keeps leaders up at night — from poor data quality to PII reidentification.
- The 3 main challenges AI poses — including the unique risks AI introduces and the misguided compliance efforts that put your organization at greater risk.
- Best practices for addressing the risks — like applying a unified compliance framework and eliminating security exceptions.
- What to look for in a data solution — like cloud-native scalability, irreversible data masking, and more.
Achieving AI Maturity: The Slingshot Effect
If you've ever watched a movie about space travel, you probably know about the "slingshot effect." This is where a spacecraft uses a celestial body (like the moon) as a "gravity assist" to whip them through space faster than possible with engines alone.
Advancing through the AI maturity curve works like this. Once you hit that data governance phase and ensure that your business and users are protected, the momentum becomes undeniable. Organizations that build strong data pipelines that they are free to use without fear of compliance violations will achieve incredible acceleration in their journey to the stars.
Every industry has an opportunity to remake their worlds with AI technologies. Here are some questions to ask when you look at your internal AI initiatives to determine your organization’s level of AI maturity:
Are you applying AI to a practical, internal project with a clear target benefit? You are operating at Level I.
Are you layering AI throughout your business’s operations? Is it improving user experience, growth, revenues, or earnings? You are operating at Level II.
Is AI driving deep insight and change for your organization? Is it enabling you to create solutions that will redefine your industry? You are operating at Level III.
If you haven't started utilizing AI programs, you are at Level 0 and falling fast behind the rest of the world.
In 10 years, the leading organizations in nearly every industry will have taken full advantage of AI technologies to revolutionize their industry and solidify their positions.
Embrace the AI Maturity Model with Proactive Data Compliance
As your enterprise progresses through the AI maturity model, compliance and data security become increasingly important. Organizations that prioritize AI compliance at each stage of their maturity journey now will be better positioned for success. Those who ignore data compliance risk serious problems like hefty fines, data breaches, and AI model failures.
Deliver AI-Ready Compliant Data at Scale
Perforce Delphix provides comprehensive solutions for AI and analytics that support your organization’s progression throughout the AI maturity curve. We help ensure that secure, compliant, and high-quality data powers your AI initiatives. Delphix lets you:
Protect 170+ data sources, including Microsoft Fabric, Microsoft Azure, Snowflake, and Databricks.
- Mask up to 4 billion rows per hour, eliminating costly delays and speeding up innovation.
- Fully integrate with AI workflows across on-premises and cloud platforms, ensuring effortless compatibility with AI pipelines.
- Ensure consistent masking across hybrid cloud environments, maintaining data accuracy and improving adoption by analysts and developers.
Build the strong foundation needed to accelerate your journey from basic automation to strategic integration and ultimately, transformational AI. Contact us to discuss how we can help your organization speed up compliance, enhance data security, and speed up your AI-fueled innovation.
Contact AI & analytics experts
This blog was originally authored by Jedidiah Yueh and was updated by Steve Karam.